Intelligent Automation
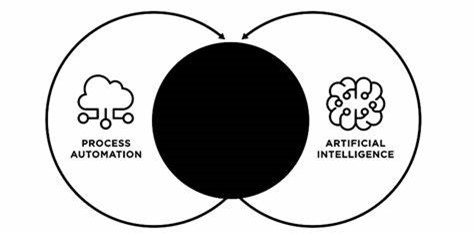
Intelligent automation is the combination of artificial intelligence (including natural language processing, machine learning, autonomics and machine vision) and automation. As a result, Intelligent Automation is defined as the fusion of these “smart” technologies with automated processes to improve business process automation.
Natural Language Processing: Natural language processing (NLP) is an Artificial Intelligence (AI) subfield (AI). This is a widely used technology for personal assistants in a variety of fields and industries. This technology analyses the user’s speech, breaks it down for proper comprehension, and processes it accordingly. This is a relatively new and effective approach, as a result of which it is in high demand in today’s market. Natural Language Processing is a new field that has already seen many advancements, such as compatibility with smart devices and interactive human conversations. AI applications in NLP focus on knowledge representation, logical reasoning, and constraint satisfaction. It was first applied to semantics and then to grammar in this case. The widespread use of statistical approaches such as machine learning and data mining on a massive scale has resulted in a significant change in NLP research over the last decade. Because of the amount of work that needs to be done these days, the need for automation is never-ending. When it comes to automated applications, NLP is a very beneficial aspect. NLP’s applications have made it one of the most in-demand methods for implementing machine learning. Natural Language Processing (NLP) is a field that studies how computers and humans communicate in natural language by combining computer science, linguistics, and machine learning. The goal of natural language processing (NLP) is for computers to be able to understand and generate human language. This isn’t just for show.
Machine learning: Machine learning is a crucial part of the rapidly expanding field of data science. Algorithms are trained to make classifications or predictions using statistical methods, revealing key insights in data mining projects. Following that, these insights drive decision-making within applications and businesses, with the goal of influencing key growth metrics. As big data expands and grows, the demand for data scientists will rise, necessitating their assistance in identifying the most relevant business questions and, as a result, the data needed to answer them.
Machine vision: Machine vision is a tool used in the industrial field to control machines autonomously. Computer vision is a type of technology that allows images to be processed and understood. Consider an industrial robot that is specifically equipped and programmed
to detect faulty products on a manufacturing line. While computer vision focuses on the algorithms that identify visual defects, a machine vision system encompasses the entire system that both detects and eliminates defects from the manufacturing process.
Automation: Electronics and computer-controlled devices are being used to replace manual operations.
Artificial intelligence and automation are not new concepts, but they have advanced significantly in recent years. Machine learning techniques, sensor improvements, and ever-increasing computing power have all contributed to the development of a new generation of hardware and software robots with practical applications in nearly every industry sector.
Intelligent automation systems detect and generate massive amounts of data, and they can automate entire processes or workflows while learning and adapting as they go. From collecting, analysing, and making decisions about textual information to guiding autonomous vehicles and advanced robots, applications range from routine to revolutionary. It is already assisting businesses in going beyond traditional performance tradeoffs to achieve unprecedented levels of efficiency and quality.
lately, most robotics applications were found in the primary sector, automating and removing the human element from the production chain. Its first foray was into replacing menial tasks, and many organizations integrated robotics into their assembly line, warehouse, and cargo bay operations. Businesses in the tertiary sector are already using new technologies and the robotic paradigm to automate processes and replace humans in low-value-added tasks.
We can gain the following advantages from using Intelligent Automation,
- increase process efficiency (Increase the speed of your processes while maintaining a high level of quality).
- Make the most of bots (Rather than relying on RPA (Robotic Process Automation) bots to complete tasks on their own, combine them with other technologies to achieve intelligent automation).
- Release employees from repetitive tasks (With intelligent technologies assisting in making informed decisions, you can be sure that routine tasks are being completed fully, effectively, and error-free).
- Interpret Big Data (Large amounts of data that would take humans a long time to manage can be handled and interpreted by intelligent automation).
- Reduce costs (Automating tasks can save the equivalent of one or more full-time employees, who can then devote their time to higher-value tasks or be deployed elsewhere in the company).
- Improve governance and fraud detection (You can ensure that governance is followed to the letter, and intelligent systems can detect and prevent fraud by identifying suspicious activity).
Intelligent automation can benefit businesses, particularly in processes where data needs to be managed, moved from one part of the business to another, or presented to a client, such as automating customer requests. These tasks would take much longer and be more prone to errors if they were not automated intelligently.
These technologies are becoming more widely available as open-source or low-cost products, as well as cloud-based services. However, they can only replace humans in tasks that are not central to the processing platform. If they decide to replace it, they should carefully choose a suitable solution that can be easily integrated using a service layer. They will have to adapt the processes to the new system rather than the new system to the old way of working to get the most out of the new system in terms of automation. if they choose to overhaul it, they should componentize the architecture, factorize the elements, review the process, isolate, simplify, and reduce the transactional platform to its core, and introduce a secure yet high-performance service layer to integrate it with peripheral systems and IA technologies.
IA technologies can and will be used in a variety of process areas, but there will almost certainly be domains where software cannot replace humans, such as deal structuring, where a significant amount of creativity or intelligence is required. When regulations mandate that a human is in charge of the financial review process, IA can assist but not replace humans. Obviously, it will not completely replace humans, as customers will flee if they can only interact with machines – or perhaps it is just a matter of attitudes and time.
Conclusion:
- Artificial intelligence-based automation is made possible by a combination of new types of software and recent advances in computing power.
- Technologies such as machine vision, speech recognition, natural language processing, machine learning, and autonomics can be combined to automate processes by interpreting facts, making decisions, and adapting to change.
- These technologies are still in the early stages of development, but they are already capable of replacing humans in a variety of tasks.
- To get the most out of this technology, legacy core platforms will almost certainly need to be overhauled or replaced from the ground up.