Prof. Yuzhe Ma from IEEE CEDA Guangzhou Won Best Paper Award at 2023 MLCAD Workshop
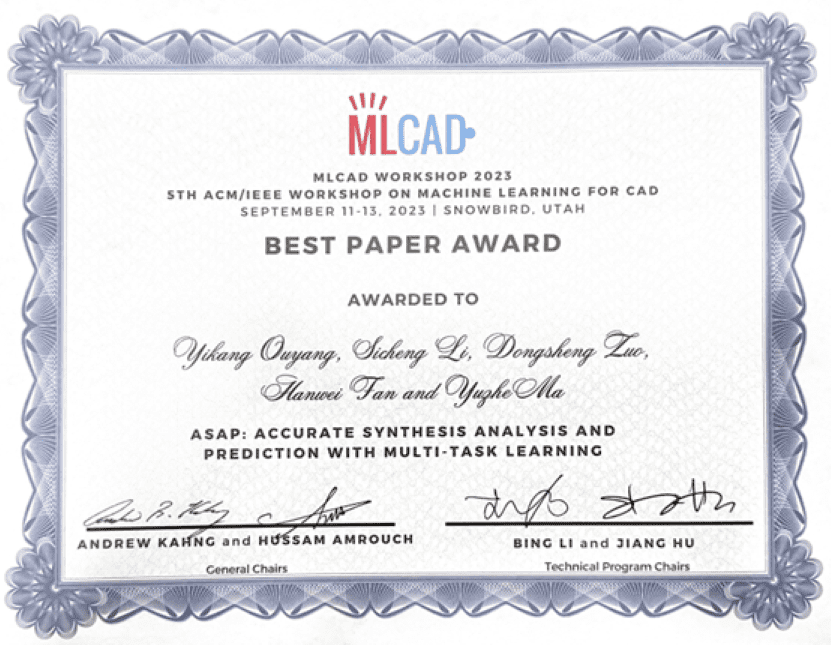
At the 2023 ACM/IEEE Workshop on Machine Learning for CAD (MLCAD Workshop), Professor Yuzhe Ma and his doctoral students Yikang Ouyang and Dongsheng Zuo were awarded the Best Paper Award. The workshop, which began in 2019, focuses on the application of machine learning (ML) technology in computer-aided chip design and electronic system design. It is jointly organized by the ACM Special Interest Group on Design Automation (SIGDA) and the IEEE Council on Electronic Design Automation (CEDA).
The fifth MLCAD Workshop was held on September 11-13, 2023, in Snowbird, Utah, USA. On September 13, the Best Paper Award and Best Student Paper Award were announced, recognizing the most innovative research contributions to the field. Professor Ma and his students won the Best Paper Award with their paper titled ASAP: Accurate Synthesis Analysis and Prediction with Multi-Task Learning, which impressed the judges with its novel approach to chip design automation. The research was conducted in collaboration with Alibaba DAMO Academy, highlighting the importance of industry-academia partnerships in advancing ML for CAD.
The MLCAD Workshop has become a vital platform for researchers and professionals to share ideas and stay up-to-date with the latest developments in ML for CAD. The Best Paper Award serves as a testament to the workshop’s commitment to promoting innovation and collaboration in this field.
Abstract of this paper
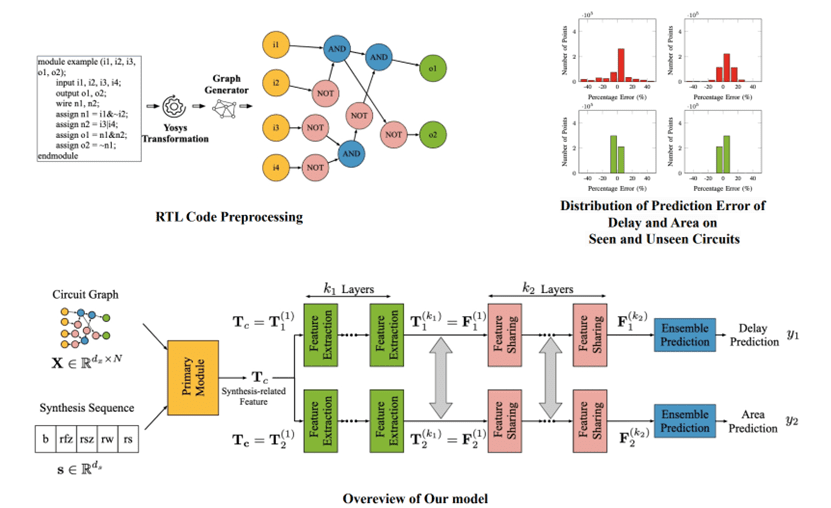
As the scale of circuits continues to grow, the design time has also increased significantly, hindering the efficient chip development process. To improve chip design efficiency, accurate estimation of circuit metrics needs to be provided to designers in the early stages of design. Inspired by the coupling relationship of circuit metrics before the logic synthesis stage, this article proposes the use of multi-task learning (MTL) to model the correlation of circuit metrics and simultaneously predict the circuit delay and area after logic synthesis.
Experimental results show that our method has a low average absolute percentage error (MAPE) of 6% on 780,000 data points tested, which is about three times better than the results without considering the coupling relationship model. In addition, we further validate that the proposed MTL model can facilitate exploration of the circuit design space, finding Pareto-optimal circuit designs in a multi-objective design space, achieving a hyper-volume improvement of 5% to 40%.

First author
Yikang Ouyang, an IEEE student member, is a second-year doctoral student in the Microelectronics Thrust of the Hong Kong University of Science and Technology (Guangzhou). He obtained his undergraduate degree in microelectronics from Sun Yat-sen University in 2022. His main research interests include electronic design automation (EDA) algorithms and machine learning-assisted circuit design.
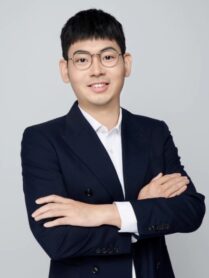
Advisor
Professor Yuzhe Ma is one of the founding members of IEEE CEDA Guangzhou and currently serves as the Vice Chairman of IEEE CEDA Guangzhou. He is also an assistant professor and doctoral supervisor at the Microelectronics Thrust of the Hong Kong University of Science and Technology (Guangzhou). Previously, he worked as a senior research scientist at Huawei Hong Kong Research Center. He obtained his Ph.D. degree in the Department of Computer Science and Engineering at the Chinese University of Hong Kong in 2020, and his bachelor’s degree in Microelectronics from Sun Yat-sen University in 2016. His research interests are on the cross-disciplinary study of electronic design automation and machine learning, including agile VLSI design methodologies, machine learning-aided VLSI design, and hardware-friendly machine learning. Professor Ma has received Best Paper Awards from MLCAD 2023, ICCAD 2021, ASPDAC 2021, ICTAI 2019, Best Paper Award Nomination from ASPDAC 2019, and Best Research Award from 2020 ASPDAC Student Research Forum.
Personal homepage: https://facultyprofiles.hkust-gz.edu.cn/faculty-personal-page/MA-Yuzhe/yuzhema