New Approaches to Distributed State Estimation, Inference and Learning with Extensions to Byzantine-Resilience, Dr. Aritra Mitra, 17th July 2020
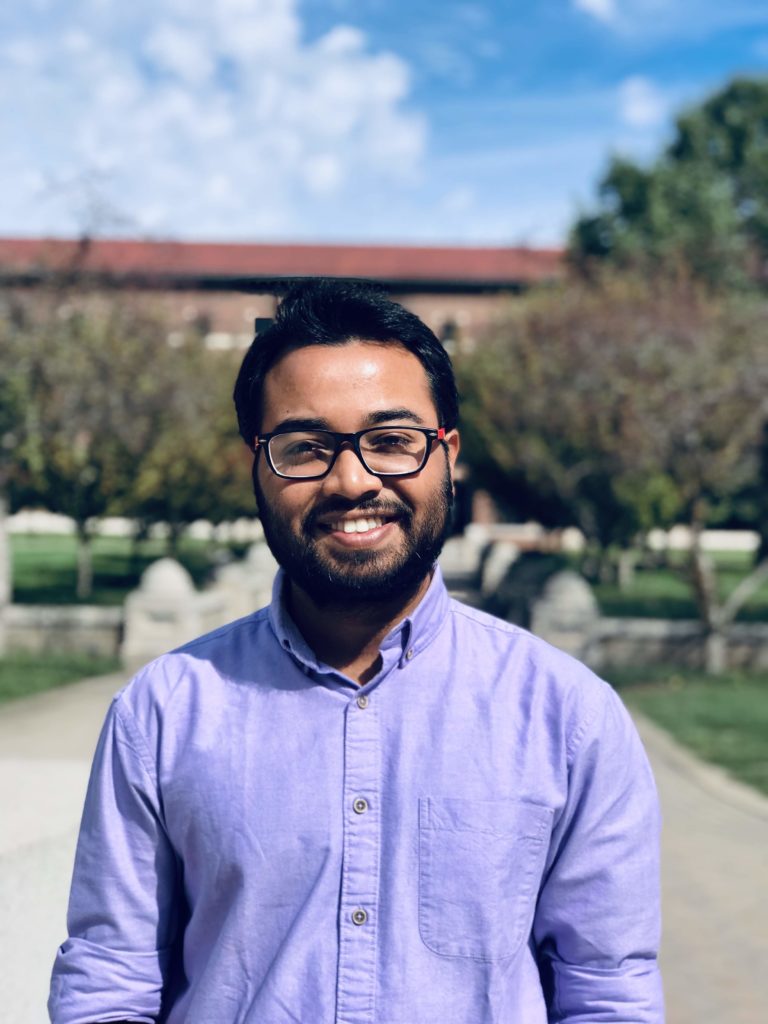
Speaker Bio: Aritra Mitra received the B.E. degree from Jadavpur University, Kolkata, India, and the M.Tech. degree from the Indian Institute of Technology Kanpur, India, in 2013 and 2015, respectively, both in Electrical engineering. He completed his Ph.D. degree in Electrical engineering at the School of Electrical and Computer Engineering, Purdue University, West Lafayette, IN, USA in July 2020. His current research interests include the design of distributed algorithms for estimation, inference and learning; networked control systems; and secure control. He was a recipient of the University Gold Medal at Jadavpur University, and the Academic Excellence Award at IIT Kanpur.
Abstract: Decision-making problems in large-scale social and engineered systems often require a network of (mobile) agents to cooperatively understand the state of the world based on local observations, and the information acquired from neighbors. Typical examples include environmental monitoring or surveillance using mobile robot teams, and learning about social or political events by a community of individuals. Identifying the underlying state in these settings is, however, fraught with several challenges: intermittent or partially informative observations of the system state, loss of communication links due to mobility and packet drops, and the potential for misbehavior by some of the agents. In this work, we address these challenges for two canonical problems, namely distributed state estimation and distributed inference/ hypothesis testing. We first propose a simple method to design distributed observers for the most general category of LTI systems, linear measurement models, and static graphs by introducing the idea of a multi-sensor observable decomposition. Exploiting the notion of age-of-information in wireless networks, we show how our approach can be extended to accommodate a broad class of time-varying graphs. For solving the distributed hypothesis testing problem, we propose a learning rule that differs fundamentally from existing approaches, in that it does not employ any form of “belief-averaging”; instead, agents interact based on a min-protocol. We prove that with probability one, each agent learns the true state exponentially fast at a network-independent rate that strictly improves upon existing rates. Finally, we describe variants of our algorithms that are resilient to adversarial agents who deliberately inject misinformation into the network, or deviate from the prescribed protocols.