Bio-inspired Computing with Memristors
The rapid development in the field of artificial intelligence has relied principally on the advances in computing hardware. However, their system scale and energy-efficiency are still limited compared to the brain. Memristor, or redox resistive switch, provides a novel circuit building block that may address these challenges in neuromorphic computing and machine learning. In this talk, I will first briefly introduce the promises and challenges with regards to the use of memristors in realizing bio-inspired computing. Secondly, I will show examples of memristor-based neuromorphic computing. Novel memristors have been used to simulate certain synaptic and neural dynamics, which led to prototypical hardware spiking neural networks practicing local learning rules and reservoir computing. Thirdly, I will discuss the 128×64 1-transistor-1-memristor array for hardware accelerating machine learning. This prototypical processing-in-memory system implemented deep-Q reinforcement learning for control problems, as well as supervised training of convolutional and/or recurrent networks for classification.
Registration URL: https://hkust.zoom.us/meeting/register/tJYsduysrD8sGtZ960QL9iVm40stk5zrvqec
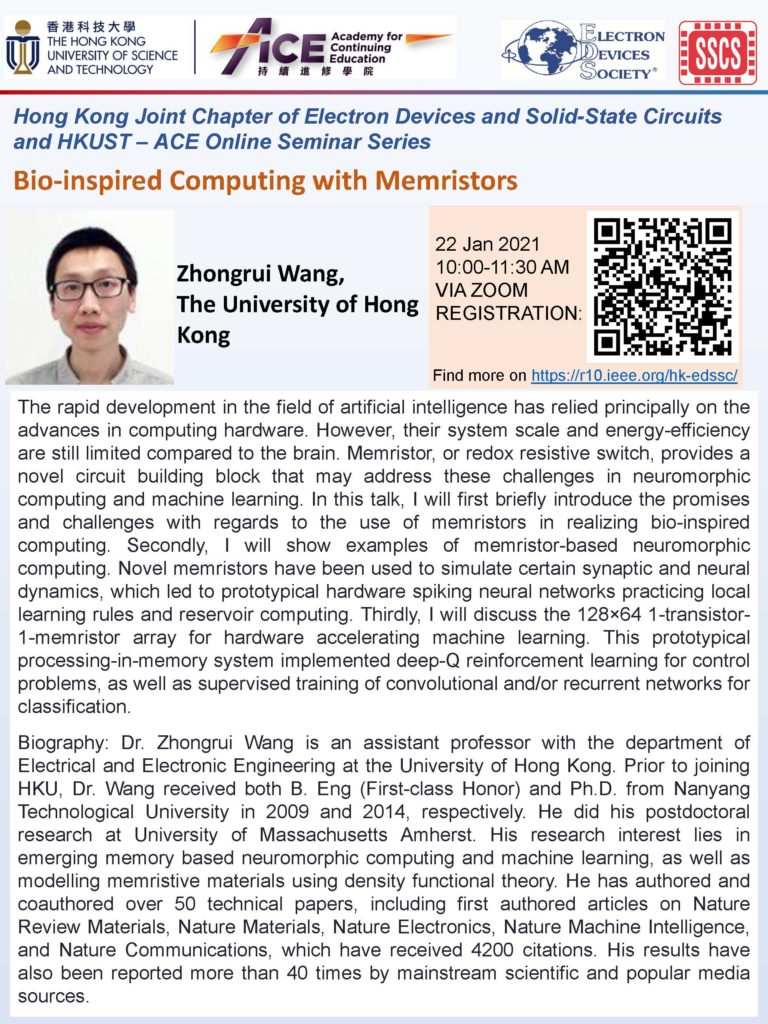